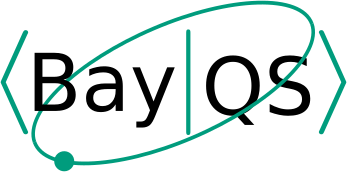
Quantum computers have the potential to bring about disruptive change in many industries and to facilitate a wide range of new applications. Examples of these applications include efficient simulations for the development of catalysts, enzymes and drugs in the chemical and pharmaceutical industries; the simulation of new materials to make solar cells and batteries more efficient in the energy and automotive sectors; efficient solutions for complex optimization problems in the logistics, finance and insurance industries; image and signal processing for medical and industrial X-ray computed tomography applications; mobile communications and — of course — applications in artificial intelligence and cybersecurity.
While the development of quantum computers is proceeding at a rapid pace, the use of such computers in industrial applications is still in its early stages. For quantum computing to be widely applicable, the relevant foundations need to be laid to make it trustworthy and easier to use.
This is the goal of BayQS — the Bavarian Competence Center for Quantum Security and Data Science — where researchers are studying relevant software issues in the context of quantum computing.
BayQS is developing solutions to help the industry identify the advantages that quantum methods offer for practical problems, while also minimizing risks in relation to the intellectual property rights to the research results obtained when using the quantum hardware access that is currently available. At the same time, solutions are being designed to simplify the development of quantum algorithms.